Why Information Governance is Critical to the Success of Smaller, Nimbler AI Models
- Max Rapaport
- Jul 9, 2024
- 3 min read
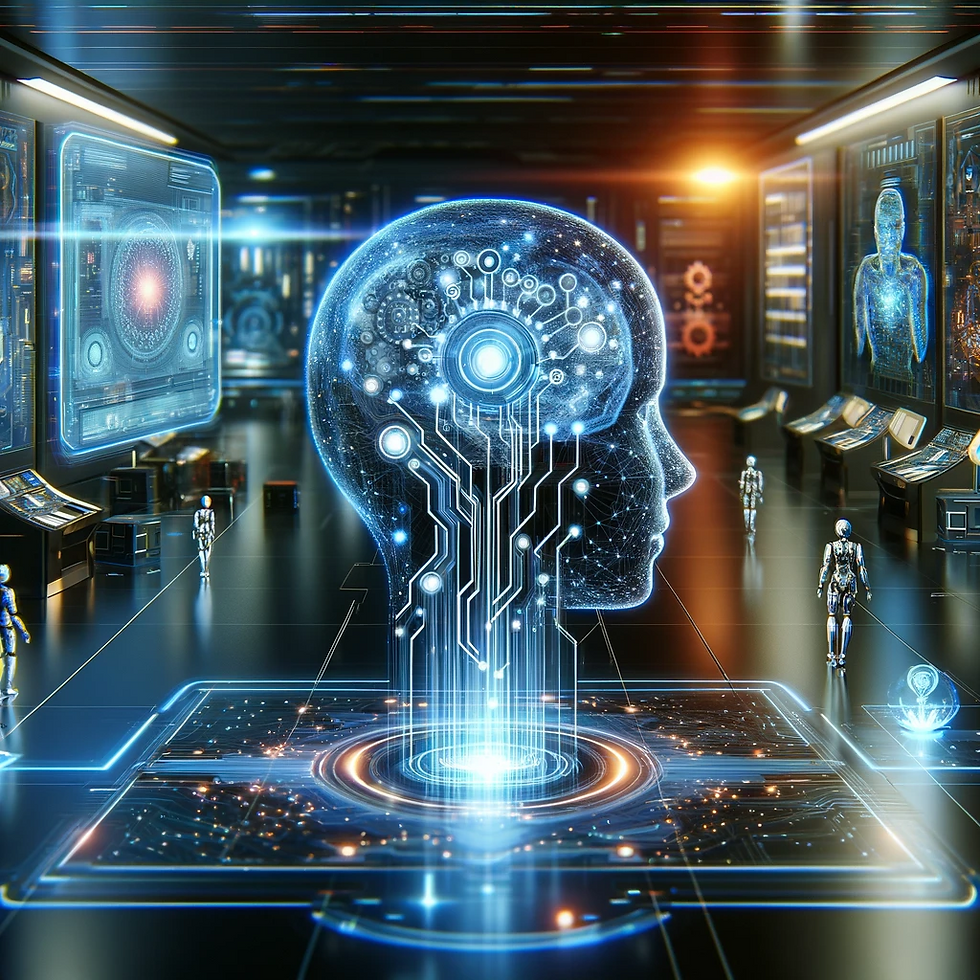
The AI industry has witnessed a shift from developing enormous, all-encompassing models like OpenAI's GPT-4, which boasts over a trillion parameters, to smaller, more agile models. These smaller models are designed to perform specific tasks efficiently and at a fraction of the cost. Smaller models also offer advantages in speed and efficiency. Perhaps most importantly, can be deployed on local devices, eliminating the need for constant cloud connectivity, which is a significant advantage for applications requiring cost-effective, real-time processing and privacy.
In one example, Microsoft’s Phi models are only 1/100th the size of GPT-4 yet are capable of performing many tasks nearly as well. According to Microsoft, the Phi series models reduce computational costs significantly, making them ideal for applications that do not require the extensive capabilities of large models. Another example can be seen in Apple’s plans to use small models to run AI software entirely on phones, making it faster and more secure.
Due to their lower cost and carbon footprint and more specific focus, smaller, specialized AI models can offer organizations a compelling alternative to their massive counterparts. However, to properly realize their benefits, their deployment must be governed by robust information governance practices.
Information governance encompasses the policies, procedures, and standards that ensure data is managed effectively, securely, and ethically throughout its lifecycle. According to leading information governance authority ARMA International, IG encompasses the “structures, policies, procedures, processes, and controls implemented to manage information at an enterprise level, supporting an organization’s immediate and future regulatory, legal, risk, environmental, and operational requirements." This definition highlights the comprehensive nature of information governance, emphasizing its role in integrating various information management disciplines to ensure that organizational information is managed effectively, securely, and compliantly.
As illustrated below, IG best practices such as rigorous version control, regularly deleting “junk” data and obsolete records, and engaging in data auditing and validation are critical to the successful deployment and continued adoption of smaller-scale and more focused AI models.
First, the highly focused nature of these models combined with their smaller data footprint creates a scenario in which each piece of data fed into the model is much more important than it would be within a large model like Chat GPT. Practically, this means that small-scale AI models are much more highly dependent on data quality than their larger counterparts and have a heightened need for clean, accurate, and well-curated datasets to function effectively. Conversely, poor data quality can lead to inaccurate predictions and unreliable outputs. Implementing rigorous data validation protocols and maintaining comprehensive metadata standards ensure that only high-quality, accurate data is fed into smaller AI models. By systematically auditing data sources and enforcing data quality rules, organizations can prevent errors and inconsistencies within these models.
Another unique trait of many smaller-scale AI models, particularly those deployed by highly regulated organizations such as banks and healthcare providers is the need to ensure that their AI models comply with regulatory standards, and in particular, data privacy standards. By systematically eliminating expired records and junk data, information governance frameworks help organizations navigate complex regulatory landscapes and implement necessary safeguards. Moreover, these safeguards are increasingly mandated by law. For example, the European Union’s AI Act emphasizes the need for rigorous AI model risk management and third-party risk scrutiny, highlighting the importance of governance in AI deployments.
Finally, taking an information governance-first approach to smaller-scale AI model development is critical to developing trust. Users and stakeholders need to be confident that AI models are unbiased, transparent, and fair. Information governance practices, such as data lineage tracking and bias audits, help to ensure that AI models are not only effective but also ethical. According to a Salesforce survey, nearly 70% of workers are hesitant to adopt AI if they do not trust the data that trains it. By ensuring that these models operate within strict privacy and security frameworks, organizations can enhance both the performance and user trust in their AI applications.
The successful future of AI lies not just in the scale of the models but in their specialization and efficiency. Smaller AI models offer significant advantages in terms of cost, speed, and accessibility. However, their success is inextricably linked to robust information governance practices. Ensuring data quality, regulatory compliance, and ethical standards are met is essential to unlocking the full potential of these models. As AI continues to integrate into various aspects of our lives, the role of information governance will only become more critical in ensuring that these technologies are reliable, secure, ethical, and worthy of users' trust.
댓글